Rubin LSST-France, LPNHE, 28-30/11/2022
Amphithéâtre Charpak
Laboratoire de physique nucléaire et des hautes énergies (LPNHE)
The next biannual meeting of the Rubin-LSST France community will be held 28-30 November 2022, at LPNHE, located at the Jussieu campus in Paris.
Details
- Meeting begins at 1:30pm on Monday 28 November.
- Meeting ends around 4pm on Wednesday 30 November.
- A meeting dinner will be organized on Tuesday 29 November (information to come).
Information about talks:
- Language: Acknowledging that some participants may not understand/speak French, using English is strongly encouraged.
- Speakers: please upload your slides on this website prior to your talk.
Remote attendance
- It will be possible to follow the meeting over Zoom, but please only register for in-person attendance.
- The Zoom link is https://u-paris.zoom.us/j/83869875817?pwd=UFpXVGNmSjhpdVI2bEphWUYxRUQ4Zz09.
Campus information
- Some recommendations for lunch.
- A map of the campus (see below as well).
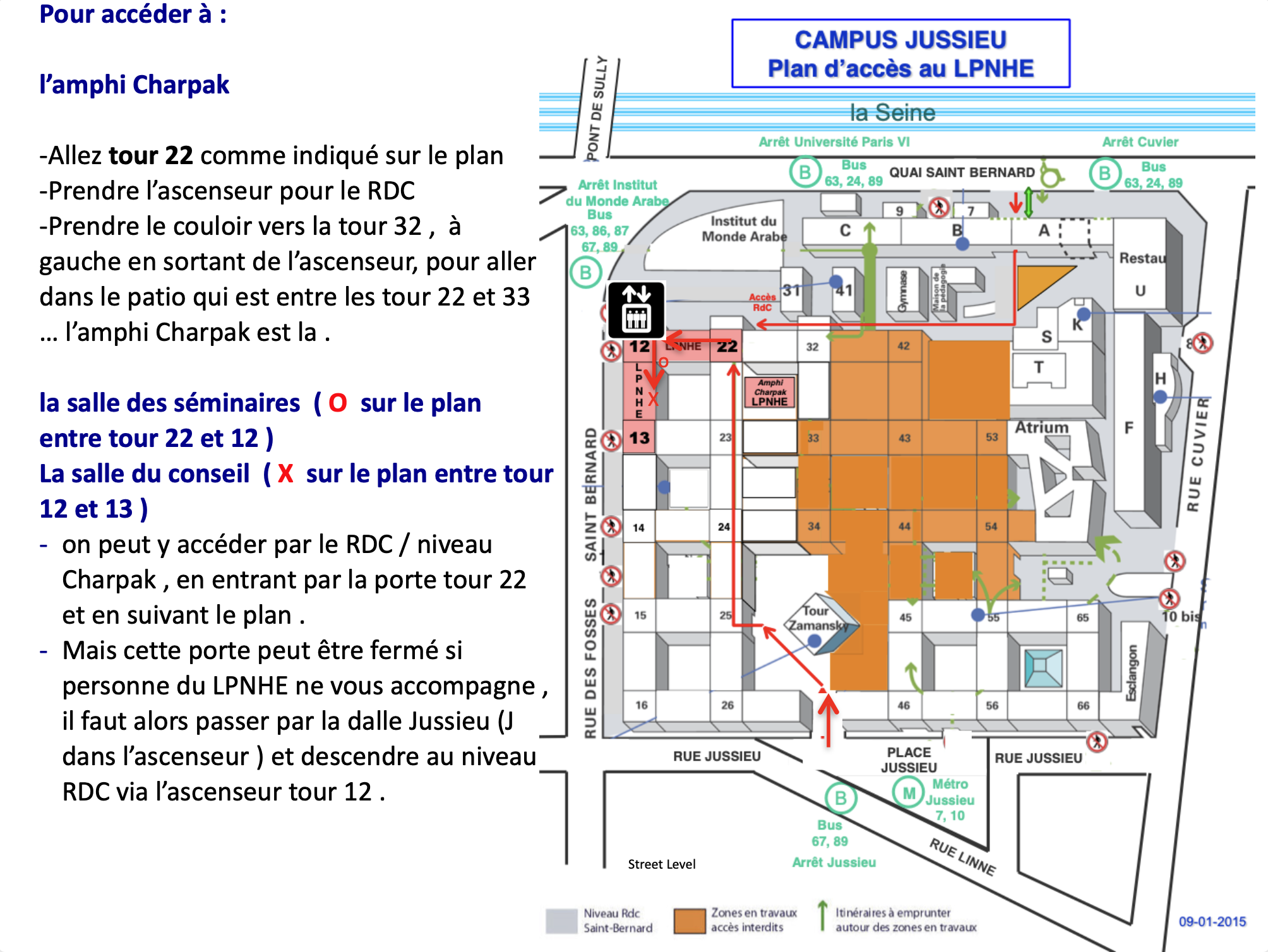
The agendas of the most recent editions of this meeting can be found online:
-
-
General news and updates: News from the Rubin Project and DESC Amphithéâtre Charpak
Amphithéâtre Charpak
Laboratoire de physique nucléaire et des hautes énergies (LPNHE)
4 Place Jussieu, Tour 22, 1er étage, 75005 ParisPrésident de session: Cyrille Doux (LPSC)-
1
Bienvenue au LPNHEOrateur: Pierre Antilogus (LPNHE)
-
2
Project and camera updateOrateurs: Claire Juramy (LPNHE/CNRS), Pierre Antilogus (LPNHE)
-
3
Communication/EPOOrateur: Gaelle Shifrin (Centre de Calcul de l'IN2P3)
-
4
DESCOrateurs: Celine Combet (LPSC), JOHANN COHEN-TANUGI (LUPM, Université de Montpellier)
-
5
LSST@Europe summaryOrateurs: Alexandre Boucaud (APC / IN2P3), Cécile Roucelle (APC)
- 6
-
1
-
15:00
Coffee break Amphithéâtre Charpak
Amphithéâtre Charpak
Laboratoire de physique nucléaire et des hautes énergies (LPNHE)
4 Place Jussieu, Tour 22, 1er étage, 75005 Paris -
General news and updates: Computing Amphithéâtre Charpak
Amphithéâtre Charpak
Laboratoire de physique nucléaire et des hautes énergies (LPNHE)
4 Place Jussieu, Tour 22, 1er étage, 75005 Paris-
7
Computing news and tools for data analysisOrateurs: Dominique Boutigny (LAPP), Fabio Hernandez (CC-IN2P3), Gabriele Mainetti (CC-IN2P3), Quentin Le Boulc'h (CC-IN2P3)
-
7
-
Science talks: Observing strategy, PFS and symbolic regression Amphithéâtre Charpak
Amphithéâtre Charpak
Laboratoire de physique nucléaire et des hautes énergies (LPNHE)
4 Place Jussieu, Tour 22, 1er étage, 75005 ParisPrésident de session: Pierre Antilogus (LPNHE)-
8
LSST Observing Strategy: status and plan
This presentation will include recent developments of LSST observing strategy presented during the last SCOC workshop in november. The current contours of the LSST observing strategy and the action plan for the next coming months will be shown.
Orateur: Dr Philippe Gris (LPC) -
9
PFS : a wide field spectrograph on Subaru.
PFS is a unique wide field multiplex spectrograph with a 1.3 deg$^2$ f.o.v. and covering the wavelength domain: 0.38-1.26$\mu$m. PFS will start operating mid 2024. It will perform a 360 nights survey with three components: a cosmology survey to investigate the dark sector; an archeology survey for the origin of the milky way and nearby dwarf galaxies; a galaxy evolution survey to reveal the cosmic web between $0.8 \le z\le 2$ as traced by galaxies + HI gas and explore the high redshift universe. In this talk I will present PFS and the three components of the "Subaru Strategic Program" survey.
Orateur: stephane arnouts (LAM) -
10
Multiview Symbolic Regression : learning equations behind examples
Symbolic Regression is a data-driven method that searches the space of mathematical equations with the goal of finding the best analytical representation of a given dataset. It is a very powerful tool, which enables the emergence of underlying behavior governing the data generation process. Furthermore, in the case of physical equations, obtaining an analytical form adds a layer of interpretability to the answer which might highlight interesting physical properties.
However equations built with traditional symbolic regression approaches are limited to describing one particular event at a time. That is, if a given parametric equation was at the origin of two datasets produced using two sets of parameters, the method would output two particular solutions, with specific parameter values for each event, instead of finding a common parametric equation. In fact there are many real world applications – in particular astrophysics – where we want to propose a formula for a family of events which may share the same functional shape, but with different numerical parameters
In this work we propose an adaptation of the Symbolic Regression method that is capable of recovering a common parametric equation hidden behind multiple examples generated using different parameter values. We call this approach Multiview Symbolic Regression and we demonstrate how it can reconstruct well known physical equations. Additionally we explore possible applications in the domain of astronomy for light curves modeling. Building equations to describe astrophysical object behaviors can lead to better flux prediction as well as new feature extraction for future machine learning applications.Orateur: Etienne Russeil
-
8
-
Free work/hack/brainstorming time Amphithéâtre Charpak
Amphithéâtre Charpak
Laboratoire de physique nucléaire et des hautes énergies (LPNHE)
4 Place Jussieu, Tour 22, 1er étage, 75005 Paris
-
-
-
Science talks: Clusters and photo-z Amphithéâtre Charpak
Amphithéâtre Charpak
Laboratoire de physique nucléaire et des hautes énergies (LPNHE)
4 Place Jussieu, Tour 22, 1er étage, 75005 ParisPrésident de session: Benjamin Racine (CPPM/IN2P3/CNRS)-
11
Galaxy cluster mass reconstruction using weak lensing shear multipoles
Weak lensing is a powerful tool to estimate the matter distribution around massive galaxy clusters. In general, such effect can be measured by estimating the averaged tangential shear of background galaxies in circular annuli from the lens center. In addition to the average tangential shear, valuable informations on the underlying dark matter distribution can be extracted by using shear multipoles, sensitive to higher order moments of the projected matter distribution. By releasing the spherical hypothesis of halo dark matter density, joint analysis of shear multipoles can be used to improve weak lensing mass reconstruction of massive clusters. We use the data of the Three Hundred project, which allows to perform our weak lensing analysis for the different orientations available in the simulation. We show that using shear multipoles enables not only to have constraints on halo triaxiality, but can also improve the mass reconstruction of individual massive clusters.
Orateur: Constantin Payerne (LPSC (IN2P3)) -
12
Impact of blending on galaxy clusters using DC2 catalogs
Galaxy clusters trace the highest peaks in the density of the Universe. Therefore, their abundance is a powerful probe to constrain cosmological parameters, expansion of the Universe and give information on the growth of structures. However, since the density of galaxies is significantly higher, these latter may appear to overlap on the line of sight and have their respective fluxes blended. This effect, called blending, significantly distorts individual galaxy measurements such as shapes or photometric redshifts, hence the need to study it.
This talk will present matching algorithms used to identify blended systems from DC2 catalogs, as well as distribution and proportion of blends in galaxy clusters. The impact of blending on shear profiles and inferred cluster masses will also be discussed.Orateur: Manon Ramel (LPSC / IN2P3) -
13
Galaxy cluster detection on LSST simulated images using convolutional neural networks
The distribution of galaxy clusters, the largest gravitationally bound structures in the Universe, helps us to estimate fundamental constants and constrain different cosmological models. With the expected development and commissioning of astronomical instruments, such as LSST, in the next decade, the depth of imaging data for a significant area of the sky will allow us to select nearly complete samples of galaxy clusters at redshifts up to z~1. To test the cluster detection technique that works directly with the reduced images, we have applied the convolutional neural network Yolo v3, trained on SDSS color images for redMaPPer clusters, to precomputed color images for LSST DC2 simulation. In order to reach performance similar to that one for SDSS images we used the same filter set and color scheme for DC2 cutouts. Our results demonstrate that Yolo is well transferable and can give reliable results even applied to datasets different from those it was trained on.
Orateur: Kirill Grishin (Universite de Paris) -
14
Towards new approaches to cluster detection for cosmology
Galaxy clusters and dark matter halos constitute a building block of many cosmological analyses. However, amongst simulations and observations, there is a wide variety of definitions of what a cluster is from a physical standpoint that do not necessarily match with each other. On top of that, on the algorithmic side, detection strategies can vary greatly from traditional friend-of-friend approaches to image-based detection using convolutional neural networks. However, the potential of the latter is often hampered by the black-box aspect of it. In this talk, I will present the starting investigations on a new approach to combine the best of both worlds, using physics to guide machine learning algorithms. I will discuss in particular how the task of cluster detection may be reframed as a game on graphs that can be played with algorithms similar to AlphaGo. I will then present a road-map for such project to succeed, the challenges, and what it could bring to both numerical and observational cosmology.
Orateur: Vincent Reverdy (Laboratoire d'Annecy de Physique des Particules) -
15
Photometric redshift with Deep Learning technique: Application to HSC Deep Survey
Convolutional Neural Networks have recently shown high performances in measuring photometric redshifts in the local SDSS surveys. Extending this technique at higher redshift remains a challenge due to the lack of representativity of the training set for faint sources. In this talk we apply this technique on the state-of-the-art HSC deep imaging survey (with UgrizY photometry down to i~26.5), which mimics the future LSST survey and where a large spectroscopic redshift training set is available. With respect to previous works, we first demonstrate that a multi-modal approach allows us to better extract the features available in the multi-band images. We then show that accurate photo-z can be measured up to i~24.5 with a precision of 0.014 σMAD (normalized median absolute deviation of the residuals) and 2% of outliers. The availability of infrared bands improves these results. At fainter magnitudes we must rely on the 30-band photo-z’s from COSMOS2020 to build a representative training set. We show that variable conditions and SNRs across the HSC survey impact the photo-z and we present a domain matching framework to overcome this issue. We also present a relabeling technique to exploit the large amount of unlabeled data.
Orateur: Reda AIT OUAHMED
-
11
-
10:40
Photo de groupe Amphithéâtre Charpak
Amphithéâtre Charpak
Laboratoire de physique nucléaire et des hautes énergies (LPNHE)
4 Place Jussieu, Tour 22, 1er étage, 75005 Paris -
10:45
Coffee break Amphithéâtre Charpak
Amphithéâtre Charpak
Laboratoire de physique nucléaire et des hautes énergies (LPNHE)
4 Place Jussieu, Tour 22, 1er étage, 75005 Paris -
Parallel work sessions: Clusters Amphithéâtre Charpak (LPNHE)
Amphithéâtre Charpak
LPNHE
Président de session: Celine Combet (LPSC) -
Parallel work sessions: Photometric Corrections with Forward Global Calibration Model in the context of DM Rubin-LSST science pipeline Salle du conseil (LPNHE)
Salle du conseil
LPNHE
Président de session: Dr Sylvie Dagoret-Campagne (IJCLab) -
Parallel work sessions: ZTF Salle des séminaires (LPNHE)
Salle des séminaires
LPNHE
Président de session: Benjamin Racine (CPPM/IN2P3/CNRS) -
12:30
Lunch Amphithéâtre Charpak
Amphithéâtre Charpak
Laboratoire de physique nucléaire et des hautes énergies (LPNHE)
4 Place Jussieu, Tour 22, 1er étage, 75005 Paris -
Science focus: FINK Amphithéâtre Charpak
Amphithéâtre Charpak
Laboratoire de physique nucléaire et des hautes énergies (LPNHE)
4 Place Jussieu, Tour 22, 1er étage, 75005 ParisPrésident de session: Dr Julien Peloton (CNRS-IJCLab)-
16
Fink: status
Roadmap, overview of the status
Orateur: Julien Peloton (CNRS-IJCLab) -
17
MicrolensingOrateur: Petro Voloshyn (IJCLab/Université Paris-Saclay)
- 18
-
19
Finding active galactic nuclei through Fink
The Fink broker contains a series of machine learning based modules, which enables fast processing of the data stream. We present the Active Galactic Nuclei (AGN) module as currently implemented within the Fink broker. It is a binary classifier based on a Random Forest algorithm using features extracted from photometric light curves, including a color feature built using a symbolic regression method. Additionally an active learning step was performed to build an optimal training sample. Two versions of the classifier currently exist treating both ZTF and ELAsTiCC alerts. Results show that our designed feature space enables high performances of traditional machine learning algorithms in this binary classification task.
Orateur: Etienne Russeil -
20
Fink Pair Instability Supernovae classifier
Pair-instability supernovae (PISN) are stellar explosions marking the end of life of the first generation of stars to exist in our Universe. Despite being theoretically predicted, up to this point there are no confirmed PISN observations.
The ELAsTiCC challenge, which anticipates data to be generated by the Rubin observatory, will contain a significant amount of PISN. We present a PISN module developed for the Fink broker. It is a binary classifier based on a Random Forest algorithm using features extracted from photometric light curves. We explore two types of features, one based on a parametric fit and another constructed from statistical properties of the light curves. We investigate the efficiency of this approach in distinguishing between PISN and other classes of objects within the ELASTiCC data. We also analyse scores from applying our method test data from ELAsTiCC, and discuss necessary modifications for applying it to upcoming LSST data.Orateur: Etienne Russeil -
21
Identification of optical Orphan Gamma-Ray Burst Afterglows in Rubin LSST data with the alert broker FINK
Gamma-Ray Bursts (GRBs) are among the most energetic phenomena in the Universe. The interaction of their blast wave with the Interstellar Medium produces an afterglow that can be observed from a larger angle, in a wide range of the electromagnetic spectrum and during more time than the prompt emission. Viewed off-axis, this emission has a negligible gamma-ray flux and is hence called ”GRB orphan afterglow”. Their properties make them good candidates to learn more about the GRB physics and progenitors or for the development of multi-messenger analysis, like in the case of GW170817A. According to most theoretical models, orphan afterglows should be found as slow and faint transients. This is why the Rubin Observatory shall significantly improve their detection : thanks to its limiting nightly magnitude of 24.5 and its large field of view, it should be able to detect up to 50 orphans per year. To identify orphan afterglows in Rubin LSST data, we plan to use the characteristic features of their light curves which depends on several parameters
defined by the chosen model. In this work, we generated a population of short GRBs and simulated their afterglow light curves with the afterglowpy package using a statistical distribution for each of the studied parameters. We then used the rubin_sim package to simulate pseudo-observations of these orphan afterglows with Rubin LSST and we found that about 4% of the afterglows will be observable orphan afterglows. These results are used to study the correlations between the parameters of the GRBs afterglow model and the features of the pseudo-observed orphan afterglow light curves, and will ultimately allow us to implement a filter in the alert broker FINK.Orateur: Marina Masson (LPSC) -
22
Early supernovae Ia classification using active learning
We describe how the Fink broker early supernova Ia classifier optimizes its ML classifications by employing an active learning (AL) strategy. We demonstrate the feasibility of implementation of such strategies in the current Zwicky Transient Facility (ZTF) public alert data stream. We compare the performance of two AL strategies: uncertainty sampling and random sampling. Our pipeline consists of 3 stages: feature extraction, classification and learning strategy. Starting from an initial sample of 10 alerts (5 SN Ia and 5 non-Ia), we let the algorithm identify which alert should be added to the training sample. The system is allowed to evolve through 300 iterations. Our data set consists of 23 840 alerts from the ZTF with confirmed classification via cross-match with SIMBAD database and the Transient name server (TNS), 1 600 of which were SNe Ia (1 021 unique objects). The data configuration, after the learning cycle was completed, consists of 310 alerts for training and 23 530 for testing. Averaging over 100 realizations, the classifier achieved 89% purity and 54% efficiency. From 01/November/2020 to 31/October/2021 Fink has applied its early supernova Ia module to the ZTF stream and communicated promising SN Ia candidates to the TNS. From the 535 spectroscopically classified Fink candidates, 459 (86%) were proven to be SNe Ia. Our results confirm the effectiveness of active learning strategies for guiding the construction of optimal training samples for astronomical classifiers. It demonstrates in real data that the performance of learning algorithms can be highly improved without the need of extra computational resources or overwhelmingly large training samples. This is, to our knowledge, the first application of AL to real alerts data.
Orateur: Marco Leoni (Universite Paris Saclay) -
23
The ELAsTiCC data challenge: preparing the Fink broker for LSST
Fink is a community alert broker specifically designed to operate under the extreme data volume and complexity of the upcoming Vera C. Rubin Observatory Legacy Survey of Space and Time (LSST). It is a French-led international collaboration whose task is to select, add value and redistribute transient alerts to the astronomical community during the 10 years of LSST. The system is completely operational and currently processes alerts from the Zwicky Transient Facility (ZTF), considered a precursor of LSST. Nevertheless, there are still important differences between the two surveys in terms of data format and complexity. In order to simulate the interaction between broker systems and an LSST-like alert stream, the Extended LSST Astronomical Time-series Classification Challenge (ELAsTiCC) is being organized. In this talk, I will describe the challenge, its goals and the efforts by different groups within Fink to develop machine learning classification algorithms accurate and scalable to large data volumes. I will also describe how this experience helped shape our confidence in the Fink system and the resilience of its international community, which has developed modules/filters to search for supernovae, fast transients, microlensing, AGNs, anomaly detection and multi-class deep learning classifiers.
Orateur: Emille Ishida (CNRS/LPC-Clermont)
-
16
-
16:00
Coffee break Amphithéâtre Charpak
Amphithéâtre Charpak
Laboratoire de physique nucléaire et des hautes énergies (LPNHE)
4 Place Jussieu, Tour 22, 1er étage, 75005 Paris -
Tutorials: DASK Amphithéâtre Charpak
Amphithéâtre Charpak
Laboratoire de physique nucléaire et des hautes énergies (LPNHE)
4 Place Jussieu, Tour 22, 1er étage, 75005 Paris- 24
-
Science talks Amphithéâtre Charpak
Amphithéâtre Charpak
Laboratoire de physique nucléaire et des hautes énergies (LPNHE)
4 Place Jussieu, Tour 22, 1er étage, 75005 Paris-
25
Forward modelling the large-scale structure: field-level and implicit likelihood inference
While the next generation of surveys soon starting will generate orders of magnitude more data than previously, it is becoming increasingly clear that traditional techniques are not up to the challenge of fully exploiting the raw data. The last few years have seen vast progress in the field of probabilistic large-scale structure inference, which differs in intent from traditional measurements of statistical summaries from galaxy survey catalogues. In this talk, I will review recent methodological advances. First, I will present the field-level likelihood-based approach, which allows the ab initio simultaneous analysis of the formation history and morphology of the cosmic web. Second, I will discuss the inference of physical parameters when the likelihood is implicitly defined by a "black-box" simulator.
Orateur: Florent Leclercq (Institut d'Astrophysique de Paris)
-
25
-
-
-
Science talks: Photometric redshifts and supernovae Amphithéâtre Charpak
Amphithéâtre Charpak
Laboratoire de physique nucléaire et des hautes énergies (LPNHE)
4 Place Jussieu, Tour 22, 1er étage, 75005 Paris-
26
Cosmology with ZTF
LSST, with 100,000 SNeIa, is the future of supernova cosmology. However, LSST alone cannot constrain the equation-of-state of Dark Energy: a nearby sample is needed to anchor the Hubble diagram. With measured distances to 6000 SNeIa, and unbiased to z<0.05, the Zwicky Transient Facility (ZTF) ZTF will be the primary anchor for all cosmological analyses with LSST.
With over 3000 SNeIa already classified and useable for a cosmological analysis, I will present the latest updates on the cosmological analysis for this survey: ZTF-DR2. With this dataset near frozen, one major problem remains: moving from light-curves to cosmology.
In the context of the path forward for LSST, I will discuss the latest efforts to define, characterise and measure the properties of this state-of-the-art precursor sample, and prospects for the last major cosmological analysis prior to LSST: ZTFxDES. This joint sample, of 10,000 SNeIa to z=1, will be the state-of-the-art in SNIa cosmology until at least 2027: setting the stage for LSST-DR1.Orateur: Mathew Smith (IP2I/IN2P3/CNRS) -
27
Astrophysical biaises affecting the measure of the Hubble-Lemaître constant with SNe Ia
I will present SNe Ia standardization and its environmental dependence in the case of the ZTF DR2 sample.
Orateur: Madeleine Ginolin (IP2I)
-
26
-
Tutorials: DP0 Amphithéâtre Charpak
Amphithéâtre Charpak
Laboratoire de physique nucléaire et des hautes énergies (LPNHE)
4 Place Jussieu, Tour 22, 1er étage, 75005 Paris-
28
DP0 tutorial on survey property maps and systematics corrections
In this presentation we will see some examples of survey property maps, maps that track spatial variations concerning the imaging of the survey. We will use healsparse to visualize and manipulate them. In addition to this, we will discuss how to evaluate the impact that different observing conditions can have on data. In particular, we will see some examples of 1D relations, which are the basis of some systematic decontamination methods such as those performed for the DES-Y3 galaxy clustering analysis
Orateur: Martin Rodriguez Monroy (IJCLab) -
29
Photometric redshifts with DP0Orateur: Dr Sylvie Dagoret-Campagne (IJCLab)
-
28
-
10:40
Coffee break Amphithéâtre Charpak
Amphithéâtre Charpak
Laboratoire de physique nucléaire et des hautes énergies (LPNHE)
4 Place Jussieu, Tour 22, 1er étage, 75005 Paris -
General news and updates: Calibration and focal plane commissioning Amphithéâtre Charpak
Amphithéâtre Charpak
Laboratoire de physique nucléaire et des hautes énergies (LPNHE)
4 Place Jussieu, Tour 22, 1er étage, 75005 Paris-
30
Focal plane calibration: status and plans
Summary of focal plane calibration activities at IN2P3
Orateur: thibault guillemin (LAPP) -
31
Le télescope auxilliaire (AuxTel)
Le spectrographe du télescope auxiliaire (AuxTel) a pour mission de mesurer les paramètres de transparence de l’atmosphère en fonction de la longueur d’onde, pour en déduire des corrections de couleur de la photométrie issue du télescope principal.
Les outils en cours de développement seront brièvement décrits : production de flat-fields spéciaux, réduction de spectres, procédures de contrôle, extraction de paramètres atmosphériques, procédure de correction de couleur en fonction des types stellaires…
Un point sera fait sur le commissioning du spectrographe et les résultats obtenus avec les données prises depuis plus d’un an seront discutés.Orateur: marc moniez (LAL-IN2P3) -
32
Collimated Beam Projector status
The Collimated Beam Projector (CBP) is a new device to measure instrumental throughput of a telescope. We will detail the performance of a CBP version built at LPNHE and show that we reach a permil calibration of the CBP throughput itself thanks to a calibrated solar cell, and of the StarDice telescope. We will present news from the Rubin Obs. CBP under construction.
Orateurs: Jérémy Neveu (LPNHE), Thierry Souverin -
33
Update on CCOB Narrow Beam data taking preparation and data analysis
The CCOB narrow beam has been developed and built in the past several years at LPSC Grenoble with the goal to obtain a thin monochromatic beam of calibrated light useful to characterize the Rubin camera optic transmission and internal geometrical alignment.
I will give a brief overview of the apparatus and present the current data taking and data analysis strategy based on ray tracing simulationsOrateur: Johan Bregeon (IN2P3 LSPC)
-
30
-
12:20
Lunch Amphithéâtre Charpak
Amphithéâtre Charpak
Laboratoire de physique nucléaire et des hautes énergies (LPNHE)
4 Place Jussieu, Tour 22, 1er étage, 75005 Paris -
Parallel work sessions: Discussion about flat-fielding and/or about colour corrections with AuxTel Salle du conseil (LPNHE)
Salle du conseil
LPNHE
Président de session: Martin Rodriguez Monroy (IJCLab) -
Parallel work sessions: FINK Amphithéâtre Charpak (LPNHE)
Amphithéâtre Charpak
LPNHE
Président de session: Dr Julien Peloton (CNRS-IJCLab) -
Parallel work sessions: StarDice Salle des séminaires (LPNHE)
Salle des séminaires
LPNHE
Président de session: Marc Betoule (LPNHE) -
15:30
Coffee Amphithéâtre Charpak
Amphithéâtre Charpak
Laboratoire de physique nucléaire et des hautes énergies (LPNHE)
4 Place Jussieu, Tour 22, 1er étage, 75005 Paris -
Free work/hack/brainstorming time Amphithéâtre Charpak
Amphithéâtre Charpak
Laboratoire de physique nucléaire et des hautes énergies (LPNHE)
4 Place Jussieu, Tour 22, 1er étage, 75005 Paris
-